Generative AI has emerged as a scorching topic in 2023 and the pace of related business discussions and activities is remarkable and rapidly accelerating:
- Articles about ChatGPT and other generative AI technologies appear nearly daily in all major news outlets like The New York Times and The Wall Street Journal and YouTube creators deploy new content about emerging generative AI tools and use cases every week. A recent report by Goldman Sachs suggested that generative AI could raise global GDP by 7%, a truly significant effect for any single technology.
- The most prominent technology companies have entered the race for generative AI technology dominance with a rapid series of bold announcements and product releases (i.e., AWS, Amazon Bedrock; Google, Vertex AI; Microsoft, Office 365 Copilot; Open AI, ChatGPT4; Salesforce, Einstein GPT, etc.).
- Venture capital investments in the generative AI ecosystem has accelerated, with $1.7 billion in completed deals and $10.7 billion in announced investments in Q1 2023, according to Pitchbook. Prominent new startups that have raised money to build technology components for the generative AI ecosystem include Anthropic, Cohere, Inflection, LangChain, Pinecone, and Stability.ai. Large communities of AI engineers working on open-source AI projects are also developing rapidly.
- To test the impact of generative AI technologies on operational productivity and customer engagement, large corporations across several industry verticals (i.e., finance, retail, legal, manufacturing, healthcare, professional services, etc.) are announcing new investment committments and launching pilot programs at an accelerating pace.
- Finally, discussions within the U.S. Congress on how to regulate the AI industry are intensifying.
According to PitchBook research, in the short term, growth in the generative AI market will be driven chiefly by enterprise applications. Generative-relevant use cases already present a significant enterprise opportunity, estimated to reach $42.6 billion in 2023, with natural language interfaces offering the most important market due to customer service and sales automation use cases. They also expect the market to reach $98.1 billion by 2026, a 32% CAGR, even without accounting for the potential of generative AI to expand the total addressable market of AI software to consumers and new user personas in the enterprise.
It is clear, therefore, that the emergence of generative AI technologies has accelerated the importance of digital transformation as a critical driver of value creation for both small and large companies and private equity investors.
With a focus on middle-market private equity firms and portfolio companies, this article aims to help business leaders reflect on the value creation case for generative AI, the critical decisions to make, and how to lead their organizations to take the first steps into the new AI journey.
According to Dr. Andrew Ng, a globally recognized leader in AI, many valuable AI projects are now possible, and the competition is open to both new startups and existing businesses.
Critical success factors will include the ability to generate a long tail of domain-specific application ideas leveraging proprietary business data, validate the business and technical feasibility of the proposals, engage business leaders able to navigate the unique challenges of launching and validating pilot projects and MVPs (minimally viable products), and scaling the most promising business opportunities.
To start their AI journey in 2023-2024, portfolio companies should focus on three critical steps:
- Develop an organizational AI policy.
- Assess the potential impact of AI on their businesses and update their strategic and action plans accordingly.
- Start testing and validating new opportunities linked to specific business goals when appropriate.
Private equity investors, on the other hand, should:
- Assess the potential impact of AI on their portfolios.
- Decide how much to invest in new AI applications powered by proprietary business data in the next three years and how to allocate the capital across portfolio companies.
- Evaluate how to support portfolio companies best to ensure they sharpen their strategy and action plans with regard to data and AI, build relevant knowledge and capabilities, and when appropriate, share lessons learned.
During the last ten years, enterprises have been gradually adopting AI technologies to solve various business problems including, for example, demand forecasting and inventory planning, making next-best product recommendations, improving search results, predicting customer churn and fraud risk, powering anomaly detection in data analytics, and catching product defects in manufacturing. In all those cases, AI technology has proven a valuable tool but has been working behind the scenes.
The critical difference with generative AI is its ability to create new content delivered in multiple modalities (i.e., text, audio, images, videos, computer code, etc.) and generate completely new interactive and highly engaging user experiences. As a result, the rise of generative AI has the potential to be a game-changer for many more businesses.
This technology, which allows for creating original content by learning from existing data, has the power to revolutionize industries and transform the way companies operate. By enabling the assisted or autonomous automation of many tasks traditionally done by humans, generative AI can increase efficiency and productivity, reduce costs, and open up new growth opportunities.
Businesses leveraging digital transformation and generative AI technologies will continue to gain a significant competitive advantage.
At this stage, we believe that business leaders starting this new AI journey should develop their strategies on the basis of the following four fundamental concepts:
- Foundation models ("the brain") are plentiful and easily accessible.
- Practical use cases for small and large businesses already exist that can be implemented using SaaS tools and low-code/no-code third-party platforms.
- For most businesses, opportunities will be in specific vertical applications powered by proprietary business data.
- Current LLMs (large language models) can "hallucinate" and are not yet ready for "autonomous prime time".
Generative AI models will be plentiful, easily accessible, and will continue to be relatively affordable if a competitive market structure will be preserved.
Generative AI models (also referred to as "foundation models") serve as the "brain" of generative AI applications and are a class of machine learning models usually trained on a massive amount of data to create a particular type of content (however, multimodal models capable of generating different types of content are also under development). Once trained, these models can be fine-tuned or adapted to a wide array of specific tasks, effectively serving as the "foundation" for various artificial intelligence applications.
Fine-tuning a foundation model involves training the model on a specific task using a smaller, task-specific dataset after the model has already been trained on a large, general dataset. This process allows the model to specialize in the task at hand and improve its performance. For example, if you want the model to answer questions about medical information, you might fine-tune it on a dataset of medical question-and-answer pairs.
Once the foundation model is developed, anyone can build an application on top of it using the model APIs to leverage its content-creation capabilities. Foundation models, such as GPT-3 or GPT-4, can generate human-like text, translate between different languages, summarize lengthy documents, answer questions, and even write computer code. They already power dozens of applications, from the much-talked-about chatbot ChatGPT to software-as-a-service (SaaS) content generators like Jasper and Copy.ai.
Businesses outside the technology industry can also use foundation models to develop custom applications (i.e., internal chatbots querying and summarizing internal documents or providing Q&A functionalities) leveraging their engineering team and open-source solutions like AnythingLLM or low-code/no-code tools like botpress, FlowiseAI and Stack-AI.
Foundation models are typically developed and trained by large technology companies and AI startups (i.e., OpenAI, Google, Anthropic, AI21labs, Cohere, etc.) and available as paid-per-usage services. Interestingly, several open-source projects are also emerging with foundation models that claim performances close to the best commercial models (i.e., Lit-Llama, Gorilla LLM, Orca LLM, Vicuna, etc.).
The larger models are expensive to develop and train, and usually run on a cloud infrastructure (i.e., GPT 3.5 and GPT 4 APIs or other commercial and open-source models available as managed services on AWS Bedrock and other cloud-infrastructure providers). However, there is also work already in progress toward making smaller models that can be trained more efficiently and deliver effective results for some tasks, running locally on personal computers or mobile phones and without requiring Internet access (i.e., GPT4All).
As clearly articulated in a recent article (i.e., Why AI Will Save the World) by Marc Andreessen, a highly regarded technology investor, the best market structure for generative AI models will be one in which "Big AI companies", "AI Startups", and "Open source AI projects" will be allowed to continue to build their applications and compete freely. The risk, on the other hand, is that a government protected cartel insulated from the market competition will emerge due to new regulations resulting from incorrect claims of AI risks.
Middle-market companies and most large enterprises outside the technology industry will benefit from generative AI technologies through SaaS and custom applications leveraging existing foundation models and proprietary business data.
According to Dr. Andrew Ng, a globally recognized leader in AI, entrepreneur, investor, and Adjunct Professor at Stanford University's Computer Science Department, for most companies AI opportunities will be in the application layer as solutions powered by proprietary business data to drive improvements in operational productivity and customer engagement (to watch Dr. Andrew Ng full presentation on Opportunities in AI - 2023, see here).
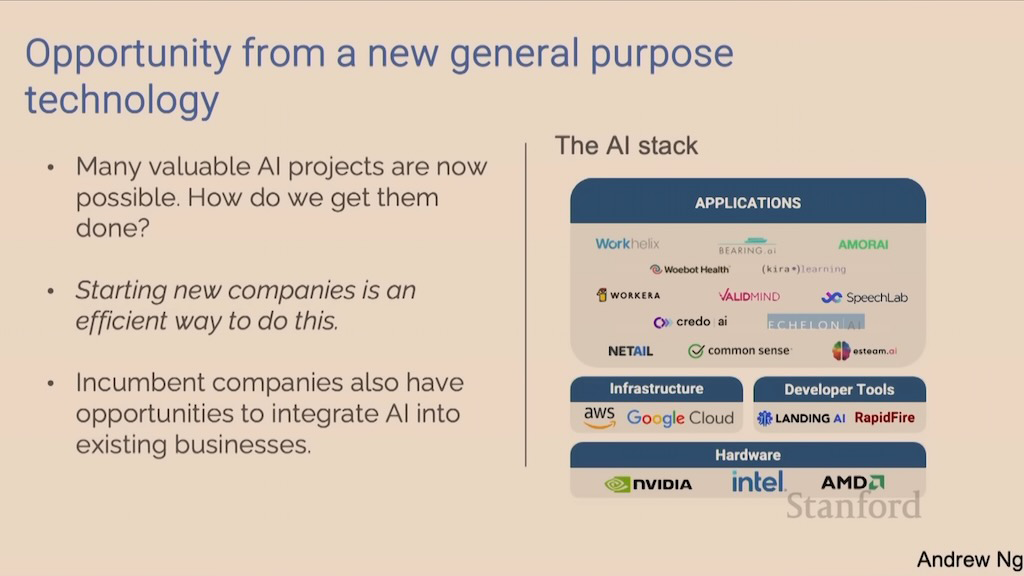
Finally, for a great introduction on what AI is and isn't, how AI models learn, and a thoughtful discussion on the expected impact of AI on jobs, I recommend reading Reprogramming the American Dream, by Kevin Scott - Microsoft's CTO.
Generative AI, particularly models like GPT-3 and GPT-4, have already shown great potential across a wide range of applications to automate, augment, and accelerate work. Based on the current state of the technology and initial pilot projects and tests, the following are some of the most significant use cases applicable across many industries:
- Content Generation: Generative AI can produce human-like text, making it useful for creating articles, blog posts, social media content, poetry, and more. It can also help with content marketing by generating creative ideas, catchy headlines, and compelling product descriptions. Leading SaaS applications for marketers include copy.ai and Jasper.
- Conversational Agents: Chatbots and virtual assistants powered by generative AI can understand and respond to user queries in a natural, human-like way. They're used in customer support, personal assistance, mental health therapy, and many other interactive applications. Several low-code/no code platforms that allow business users to develop custom chatbots already exists, including botpress, botsonic, Dialogflow, and FlowiseAI.
- Translation: Although specialized models often handle translation, generative models can also translate text between different languages, particularly when fine-tuned on translation tasks.
- Summarization: Generative AI can summarize long documents into shorter versions, which can be helpful for understanding the key points of articles, research papers, product documentation, contracts and other legal documents, financial reports and books.
- Tutoring and Education: AI-powered tutoring systems can provide personalized learning experiences, explain complex concepts, and answer student queries. To develop a better understanding about how generative AI can transform this essential field you can test Khanmigo developed by Khan Labs. Also, if you have K-12 kids, make sure to introduce them to this platform that is taking the Khan Accademy to the next level.
- Creativity and Entertainment: Generative AI is currently used to create new music, artworks, design elements, and even video game content. It's also been used to write stories, scripts, and other forms of creative writing. For a list of the top 9 music generators, see here. To explore a platform that can generate video presentations from written documents, see Creative Reality Studio from D-ID.
- Personalized Recommendations: Generative models can help create more detailed and personalized product recommendations based on user behavior, preferences, and context.
- Code Writing: Generative AI models can write code, assist in debugging, and even provide code suggestions, helping programmers to be more efficient. Popular tools include GitHub Copilot and ChatGPT Code Interpreter.
- Data Analytics: Last but not least, generative AI has the potential to significantly improve productivity in data analytics by automating various tasks and providing insights that might be difficult or time-consuming for humans to derive. Some ways generative AI can be a game-changer in the field of data analytics include the following activities (to test some of these you can use ChatGPT Code Interpreter or the Noteable ChatGPT plugin):
- Automated Reporting: Generative AI can automatically generate comprehensive reports by summarizing large datasets. These reports can include trends, outliers, and predictions, saving analysts a lot of time.
- Data Cleaning and Preprocessing: Generative AI can automatically identify and correct errors in datasets, fill in missing values, and perform data normalization. This automation can save countless hours that would otherwise be spent manually cleaning data.
- Feature Engineering: Generative models can automatically identify the most important features in a dataset and even create new features that will help in analytics or predictive modeling. This speeds up the model training process and can result in more accurate models.
- Query Generation: Generative AI can automatically create complex data queries based on simpler user inputs. This can help in retrieving more accurate and relevant data for analysis without requiring the user to have a deep understanding of query languages like SQL.
- Data Visualization: Generative AI can create insightful and complex visualizations based on the data, making it easier for analysts to understand the data and make informed decisions.
- Hypothesis Testing: By learning from the data, generative AI can formulate hypotheses or questions that a human analyst might not think to ask. It can also prioritize these hypotheses based on the likelihood of their resulting in valuable insights.
- Predictive Modeling: Generative AI can generate predictive models based on the data, which can be a starting point for analysts. While these models may not be perfect, they can often accelerate the process of creating a more refined, final model.
- Anomaly Detection: Generative models can be trained to recognize the 'normal' state of a dataset and therefore identify outliers or anomalies. This is particularly useful in fields like fraud detection, quality assurance, and network security.
- Natural Language Summaries: Generative AI can produce natural language summaries of complex data analytics results, making it easier for stakeholders who may not have a technical background to understand the findings.
These are already a rich set of use cases, and as the technology continues to improve, the potential applications of generative AI will continue to expand. Each of these use cases has the potential to create value by changing how work gets done at the activity level across business functions and workflows.
So, why is AI yet to be widely adopted?
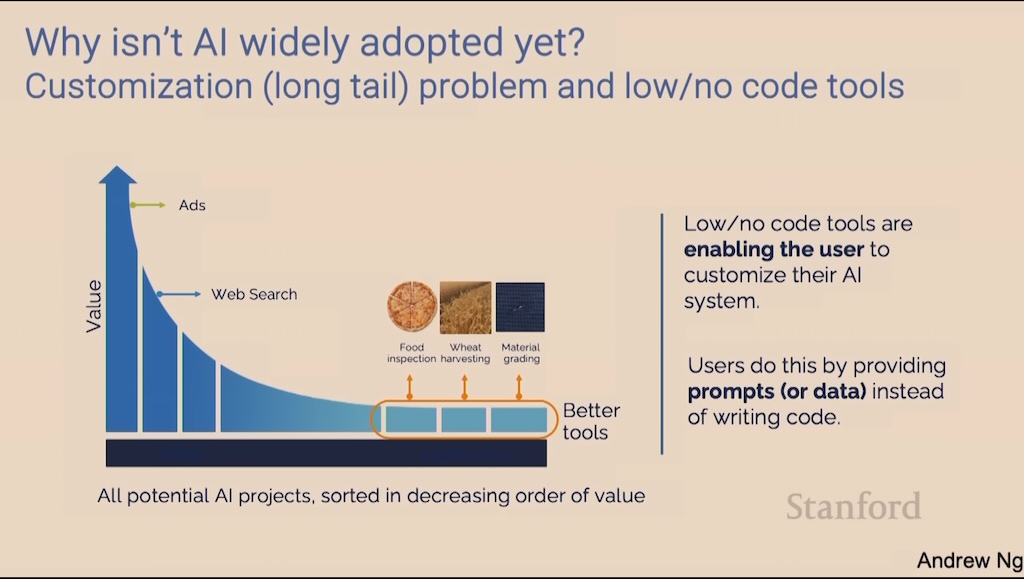
According to Dr. Andrew Ng, most of the value generated by AI will come from solutions to long-tail problems. Capturing those opportunities at scale will require:
- Involving functional domain experts currently dispersed among thousands of companies.
- Organizing and storing proprietary data in a way suitable to be ingested by AI applications.
- Low-code/no-code tools to enable businesses with no advanced software engineering skills to customize their AI systems.
For most middle-market companies, the first step into experiencing the potential of generative AI will be by testing one or more of the above use cases using third-party SaaS applications with embedded generative AI functionalities or developing simple custom applications using low-code/node-code platforms and some proprietary business data.
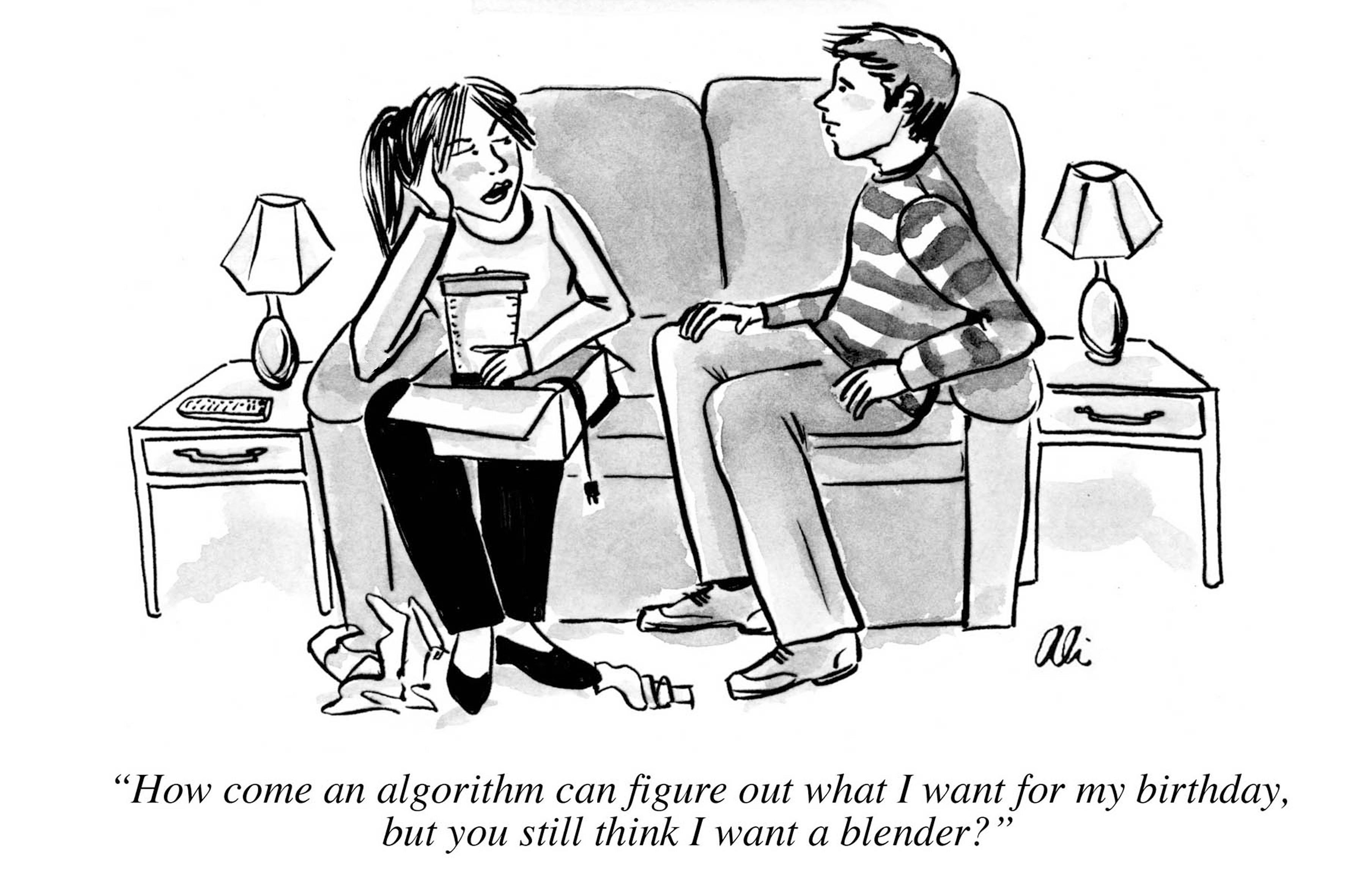
Proprietary business data is one of the most valuable assets for companies looking to leverage generative AI to drive competitive advantage. When used to train generative AI models, proprietary business data can produce insights or generate content specific to the business, provide unique solutions to the particular challenges or opportunities facing the company, produce more reliable AI models given the higher quality and relevance of the data, and generate a new form of intellectual property, adding to the company's valuation.
Examples of proprietary data that can drive competitive advantage include:
- Customer Behavior Data: Patterns of customer interactions with a website, purchase histories, and churn rates data can be used to train generative AI models to predict future customer behaviors, automate personalized marketing campaigns, or enhance the customer experience.
- Supply Chain Data: Detailed records of supplier performance, logistics costs, and inventory levels can help optimize supply chain operations, predict inventory needs, and automate procurement processes.
- Employee Performance Metrics: Employee engagement levels, productivity metrics, and performance reviews can feed AI models to predict employee attrition, help in workforce planning, and even suggest personalized employee training programs.
- Proprietary Research and Development Data: Lab results, prototype performance metrics, and product development timelines are used by companies in sectors like pharmaceuticals and technology to accelerate R&D processes and bring products to market more quickly.
- Sales and Revenue Data: Historical sales data, revenue streams by product/service, and customer lifetime value metrics allow businesses to forecast future sales, identify potential new revenue streams, and optimize pricing strategies.
- Market Research and Customer Feedback: Customer surveys, focus group feedback, and product reviews are qualitative data that can be analyzed by generative AI to automatically generate new product features, improve customer service, or even create marketing content.
- Manufacturing and Quality Control Data: Machine performance logs, quality control checks, and equipment maintenance schedules can help automate predictive maintenance and quality control, reducing downtime and improving product quality.
- Competitive Intelligence: Data gathered on competitors' pricing, marketing campaigns, and new product launches can help companies anticipate market moves and adjust their strategies accordingly.
- Financial Data: Detailed records of expenditures, income, investments, and financial ratios can help optimize models about financial risks and investment returns, and generate insights into cost-saving opportunities.
- Intellectual Property Data: Data about patents, copyrighted materials, and trade secrets is also a competitive edge and can be used to train AI models for various specialized tasks, from legal analysis to R&D optimization.
By leveraging these types of proprietary business data, companies can train more accurate and effective generative AI models to create more compelling customer experiences and continuously improve operational productivity, thereby gaining a significant competitive advantage in their respective markets.
For many companies, the next step required to capture greater benefits from new generative AI applications will be to revamp their business' data strategy and infrastructure: i.e., what data to capture, how to organize and store the data, what tools to adopt to activate the data effectively.
"Hallucination" in the context of generative AI typically refers to instances where the AI model generates information not present in or supported by the input data. In other words, the model "hallucinates" (i.e., it makes up) details or facts that are not grounded in reality.
For instance, a language model like GPT-3 or GPT-4 might produce a text that sounds plausible and well-written, but includes details, events, or facts that were not in the input or are incorrect. This can happen because the model doesn't truly "understand" the text in the way humans do; it generates responses based on patterns it learned during its training on a large corpus of text data.
AI hallucinations can sometimes be beneficial, like in creative applications where generating new, unexpected content is desirable. However, it can also lead to the spread of misinformation or produce nonsensical outputs, especially in more factual or sensitive contexts typical of many business use cases.
To mitigate this, developers are working on several strategies, such as improving the training process, using external fact-checking systems, better controlling the model's outputs, and educating users about the strengths and limitations of these AI models.
The consensus is that solutions for the "hallucination" problem will emerge soon. For now, however, the adoption of initial generative AI applications should be in supervised or copilot mode (i.e., with humans reviewing the content generated before final release), and pilot chat applications querying critical business information should be restricted to internal users only.
CEOs and private equity investors should consider exploration of generative AI powered by proprietary business data a must, not a maybe. The economics and technical requirements to start are relatively low, especially when leveraging existing SaaS tools and cloud managed-services, while the downside of inaction could be quickly falling behind competitors. On the other hand, while there is merit to getting started fast, building a basic business case first and defining a structured approach will help companies better navigate their AI journeys.
CEOs and private investors should work with their executive teams and some external advisors to reflect on where and how to play. A few initial steps to consider should include the following:
- Develop a generative AI adoption and data-sharing policy.
- Articulate an explicit business, technology, and people strategy for data and AI.
- Start testing and validating new data and AI driven opportunities linked to specific business goals.
According to a recent survey from The Conference Board, more than half of US employees already use generative AI tools, at least occasionally, to accomplish work-related tasks. Yet, some three-quarters of companies still lack an established, clearly communicated organizational AI policy.
Developing a generative AI adoption and data-sharing policy will be an essential first step for most organizations. While promoting a culture of innovation and experimentation is very important for most businesses, preserving the confidentiality of proprietary data, maintaining compliance with industry-specific regulations, avoiding sharing harmful or misleading content with customers, and maintaining focus and alignment with the business's key objectives and priorities are also essential considerations. Therefore, each organization needs to define a policy, setting some rules and guidelines to balance these critical trade-offs.
As a thought-starter, the following is a preliminary list of important questions to consider when defining such organizational AI policy:
- What functions and departments should be allowed to experiment with these new technologies freely, and which ones should not, based on potential business benefits and risks?
- Should human oversight be required for generative AI content shared with customers? How will that be implemented?
- What business data should employees be able to share with these new AI tools, and what should be restricted?
- Are there any industry-specific regulations the company must comply with, such as GDPR for data protection and HIPAA for healthcare?
- Who will be responsible for data governance, and how will the data quality be maintained?
- What training will employees need to use and manage the new AI applications effectively?
- Are there contracts, licenses, or terms and conditions that govern the data that will be shared?
- Will stakeholders and customers be informed that their data is being shared with these new AI tools? Is their consent required?
- How often will the adoption and data-sharing policy be reviewed and updated?
Given the potential transformative impact of generative AI technologies combined with proprietary business data, as a next step, CEOs and private equity investors should convene a cross-functional team of company leaders to assess its potential impact on the business, identify relevant use-cases, prioritize AI initiatives in the context of all other business priorities, and define a pragmatic course of action aligned with the overall business strategy.
Developing an explicit strategy for data and AI will benefit the company in several ways, including:
- Focusing the organization on the expected business impact by articulating how the new initiatives will benefit the company's customers, differentiate it from its competitors, and the key performance indicators (KPIs) that will be used to measure the new initiative's progress and success.
- Producing a better allocation of limited resources, including time, money, and human capital, by aligning the adoption of AI with the company's broader business objectives and strategy.
- Mitigating ethical and legal risks by setting guidelines and compliance measures to deal with the challenges that could arise from adopting AI applications (i.e., data privacy issues, risk of generating harmful, biased, or misleading content, infringement of industry-specific regulations, etc.).
- Improving stakeholder communication by providing transparency regarding the strategy goals and expected impact on both external and internal stakeholders, and accountability for the new initiatives' performance and results. AI is generating a lot of curiosity but often also fears among employees at all levels. Driving the conversation from the front will often be essential to preserve an organization energy and focus.
- Promoting operational effectiveness and efficiency by defining the human capital that will be required to execute the new strategy, appropriate plans to source and develop the required talent (i.e., hiring new people, training existing employees, building new partnerships, etc.), and considering the long-term scalability of the new AI pilot projects and the requirements to ensure their integration with existing systems and processes.
- Encouraging adaptability by providing a framework for incorporating feedback and learnings from the initial pilot programs and adapting to new advancements in AI technologies.
In summary, an explicit strategy for data and AI will provide a roadmap guiding the organization from initial testing to validation to scaling, promoting energy and excitement while aligning with business objectives, mitigating risks, and maximizing benefits. With such a strategy, companies can avoid making ad-hoc decisions that could lead to wasted resources, legal challenges, and missed opportunities.
When developing a strategy for data and AI, companies should consider a range of questions that span multiple dimensions, from business objectives to ethical considerations. Here are some critical questions to kick off the process:
- Business Objectives and Alignment
- What Are the Business Goals?: What specific business objectives are you aiming to achieve with AI? Is it to achieve a cost advantage, improve the customer experience and access to existing product and services, enhance the value of your product and services, expand your addressable market, or something else?
- How Does AI Fit into the Overall Business Strategy?: How does the adoption of AI align with the company's broader strategic goals and mission? How big is the risk of industry disruption from AI? Should your company try to lead the new wave of innovation, or should it be a fast follower?
- What is the Value Proposition?: What unique value will generative AI bring to your business, how will it differentiate you from your competitors, and what data and AI applications could drive a sustainable competitive advantage?
- Feasibility and Scope
- Is the Technology Ready?: Is AI mature enough to meet your specific business needs? What are the limitations you should be aware of? Is the required proprietary business data available in a suitable form?
- What should the Scope of Implementation be?: How should the initial scope be defined to properly test and validate the feasibility and business impact of the new opportunity? How should you define the scope of the initial MVP?
- What Resources are Required?: What financial, human, and data resources will be needed to execute the initial testing and validation phases?
- Risk Assessment and Mitigation
- What are the Ethical Implications?: How will you ensure that the AI operates within ethical boundaries, such as avoiding generating harmful, biased, or misleading content?
- What are the Legal and Compliance Risks?: Are there any industry-specific regulations or general laws (e.g., GDPR, CCPA, HIPAA) that you need to comply with?
- What is the Security Plan?: How will you protect the new AI system and the data it uses and generate from unauthorized access, data breaches, and other security risks?
- Technology Choices
- What Technology Approach will be Most Appropriate?: Can you use SaaS applications or low-code/no-code platforms for the selected use cases? Will you need to develop a custom application leveraging third-party AI APIs? Will you need to fine-tune an existing LLM (large language model)? How will you source the required technology talent?
- How Will You Manage Data?: What types of data will the AI use? How will data quality be ensured, and who will be responsible for data governance? What data infrastructure will be required to capture, store, and embed the data used by the AI applications?
- What is the Technology Stack?: What software and infrastructure will be required? What technology partners will best fit your company's requirements? How will the AI applications integrate with existing systems?
- Implementation and Operations
- Who are the Stakeholders?: Who within the organization will be involved in the design, development, and ongoing management of the new processes and applications powered by data and AI technologies?
- What is the Implementation Plan?: What are the pilot testing, validation, and full-scale implementation timelines?
- What is the Human Capital Plan?: What training will be provided to employees to ensure they can effectively understand and manage the new business processes powered by AI systems? Will new roles and responsibilities be created? What will the strategy to source the required new human capital be?
- What Stakeholder Communication will be Most Appropriate?: What elements of the new strategy should be communicated to whom and when? What level of transparency should you provide with regard to progress and achievements? Are there teams and departments that should receive special consideration?
- Monitoring and Evaluation
- How Will Success Be Measured?: What KPIs will you use to evaluate the progress and business impact of the new generative AI initiatives? What milestones will you adopt to stage capital deployment and decide which initiatives to kill and which are worth scaling?
- What is the Feedback Loop?: How will you collect and incorporate users and other stakeholders feedback to continuously improve the new business processes powered by data and AI applications?
- How Often Will the Strategy be Reviewed?: Technology and business environments are dynamic. How often will you review and update the strategy to ensure it remains relevant?
As Dr. Andrew Ng reminded us, many valuable AI projects are now possible. These projects require adapting a general-purpose AI technology to a long tail of industry-specific opportunities leveraging proprietary business data. Starting new companies will be an efficient way to pursue new ideas, but existing companies will also be able to compete by integrating AI into their existing businesses.
Existing businesses can often leverage unique advantages, including deep industry and functional domain expertise, a rich set of proprietary business data, an established customer base, and a significant network of business partners.
Middle-market private equity investors will also be in a unique position to pursue some of these new opportunities, given their unique industry and functional domain expertise, the ability to spread investments and leverage talent across several portfolio companies, and the possibility to create a central pool of expert to help portfolio companies validate the business and technical feasibility of these early projects.
Effectively pursuing these new opportunities will required an ad-hoc structured approach. Middle-market portfolio companies and private equity investors should mutuate best practices commonly used by startups and venture capital investors. The process illustrated below by Dr. Andrew Ng, that among other things is also a General Partner at the AI Fund, reflect those common best practices.
For many of these project the time (i.e., 12 to 18 months) and capital (i.e., $1-2M) required for initial testing and validation will be easily doable for many middle-market companies and private equity investors. Critical success factors will include the ability to generate a long tail of domain-specific application ideas leveraging proprietary business data, validate the business and technical feasibility of the proposals, engage business leaders able to navigate the unique challenges of launching and validating pilot projects and MVPs (minimally viable products), and scaling the most promising business opportunities.
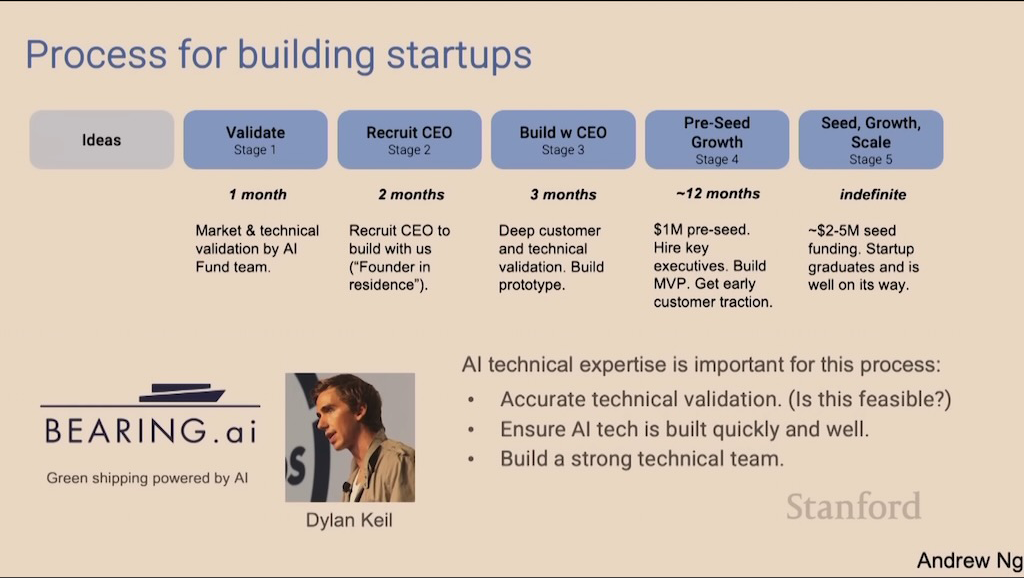
In the next three to five years, the importance of data and AI as a critical driver of value creation at many middle-market companies will continue to increase. In the coming six months, therefore, we believe middle-market private equity investors should focus on:
- Assessing the potential impact of AI on their portfolios.
- Deciding how much to invest in the coming three years to upgrade their portfolio companies data and AI capabilities and how to best allocate the available capital.
- Evaluating how to support portfolio companies best to ensure they sharpen their strategy and action plans with regard to data and AI, build relevant knowledge and capabilities, and when appropriate, share lessons learned.
Augeo Partners is a boutique firm of senior business and technology leaders with a significant track record in driving revenue growth and operational productivity through strategy, process design, digital technologies, and change management. We partner with management teams and private equity investors to accelerate value creation through a set of proven strategies and playbooks. The time to leverage digital transformation to accelerate value creation is now, and Augeo Partners can help.